The role of biomarkers in precision medicine
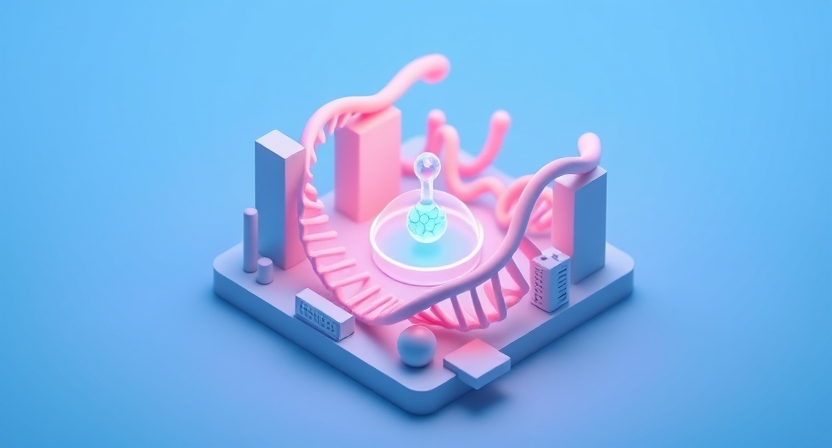
Biomarkers are used in healthcare to give objective and measurable insights into disease detection and progression. They can be used as diagnostic tools, as well as for monitoring and even predicting diseases. Biomarkers range from blood pressure levels to specific genes present in the human genome. The NCI defines a biomarker as “a biological molecule found in blood, other body fluids, or tissues that is a sign of a normal or abnormal process, or of a condition or disease”.
Types of Biomarkers
Biomarkers can be grouped into a number of different categories including diagnostic, prognostic, predictive, and monitoring. Diagnostic biomarkers are used to detect or confirm the presence of conditions or diseases. For example, the CFTR gene mutation is an identifier of cystic fibrosis. Prognostic biomarkers identify the likelihood of diseases or disease progression, such as the BRCA1/2 mutation in breast cancer. This mutation also signifies an increased sensitivity to radiation therapy, so is also a predictive biomarker. Monitoring biomarkers, such as PSA for prostate cancer, are measured repeatedly to assess the status/progression of a disease. There are also many more types of biomarkers including response, safety, multi-component, and risk.
Biological Biomarkers vs. Digital Biomarkers : which one to choose?
Biomarkers play a crucial role in disease monitoring and management. With the advancement of digital technology, a new category has emerged: digital biomarkers. Understanding their differences and combining them can optimize healthcare.
Biological biomarkers are well-established in clinical practice and provide high specificity and precision. They are clinically validated, making them reliable for tracking disease progression and enabling treatment adjustments. However, biological biomarkers are expensive due to lab work and specialized equipment, require a significant amount of bodily substances to be collected, and do not offer real-time monitoring, which can limit their effectiveness in continuous tracking.
Digital biomarkers, such as blood oxygen levels, sleep patterns, or activity levels, provide real-time, continuous and non-invasive data, making them more cost-effective. These biomarkers are increasingly popular for tracking chronic diseases like diabetes or cardiovascular conditions. They are scalable and allow for monitoring large populations, reducing the need for frequent physical visits to healthcare providers, which is useful in public health studies. Despite these benefits, digital biomarkers can have reliability issues, such as inaccurate data from sensors or improper use of devices, which may lead to skewed data and privacy concerns surrounding the collection and storage of personal health information. Furthermore, to ensure accurate results, users must follow specific guidelines, such as wearing the device correctly.
Combining biological and digital biomarkers provides a complementary approach, enabling early disease detection, proactive interventions, and personalized treatment plans. Together they improve disease prevention and management.
One size fits all vs. personalized medicine
For decades, traditional medicine used a one-size-fits-all approach, relying on broad population data to guide treatment decisions. However, as our understanding of genetics, molecular biology, and technology are shifting toward personalized medicine. This approach takes into account individual genetic, molecular, and physiological characteristics, ensuring that therapies are more effective and better suited to each patient’s unique needs.
Personalized medicine not only allows for more accurate diagnoses and targeted therapies but also leads to better patient outcomes by minimizing unnecessary treatments and side effects. By leveraging both biological and digital biomarkers, healthcare providers can create highly personalized treatment plans.For instance, in oncology, biomarkers like PD-L1 expression and tumor mutational burden (TMB) help identify which patients will benefit from immunotherapy, optimizing treatment efficacy. The integration of digital biomarkers further enhances this approach, providing real-time health data that enables dynamic, on-the-go treatment adjustments.
With the integration of digital biomarkers, this shift is further accelerated, enabling continuous, real-time insights into a patient’s health, and offering the potential for even more personalized and dynamic treatment plans.
Going further: techniques for biomarker discovery
The discovery of new biomarkers follows a structured process, moving from data collection and preprocessing. Researchers gather biological samples (e.g., blood, tissue, or genetic material) and use high-throughput technologies such as genomics, proteomics, or metabolomics to generate large datasets. At this stage, quality control ensures data reliability. In addition to traditional lab-based techniques, the application of AI and machine learning in the analysis of omics data accelerates the identification of relevant biomarkers.
Next, exploratory data analysis identifies patterns and potential biomarkers using statistical methods such as PCA and hierarchical clustering. Once candidate biomarkers are identified, advanced techniques such as CRISPR and gene-editing tools help researchers understand the biological function of genes. Machine learning techniques, such as supervised learning or unsupervised clustering methods, are used to classify samples and refine subgroups, contributing to personalized medicine.
After initial discoveries, biomarker validation is critical. Statistical methods such as Bayesian inference and survival analysis evaluate prediction accuracy. Large-scale cohort studies and clinical trials help confirm their robustness and clinical utility.
In addition, the analysis of digital biomarkers involves data science, machine learning, and AI to interpret health-related data collected from wearable devices, mobile applications, and other digital sources. AI integration, using advanced algorithms such as deep learning or neural networks, enhances the ability to process complex, real-time data, enabling more accurate predictions. These continuous insights enable early disease detection and personalized treatment adjustments, ultimately improving patient outcomes, making them a crucial tool in modern precision medicine.At Lucid Analytics, we provide expertise in every step of this process—from data collection and exploratory analysis to machine learning modeling modeling and validation. Our advanced analytics and computational capabilities empower researchers and pharmaceutical companies to accelerate biomarker discovery, ensuring faster, more accurate, and data-driven decisions in healthcare.